Data quality and privacy concerns combine to form one of the biggest perceived barriers to AI and automation integration for B2B enterprises.
There continues to be a significant concern from business leaders when it comes to confidence in their data, demonstrated in our recent Algomarketing research ‘AI & Automation Index for Global Marketing Operations’. Our findings indicate that 75% of large global B2B enterprises are delaying implementation due to data quality and bias concerns. This further evidences the paralyzing environment that can be created for marketing operations within large-scale companies when pushing to integrate algorithmic marketing techniques.
What’s more, this commonly held belief is inaccurate. Data does not need to be pristine and 100% comprehensive to unlock the benefits of AI and advanced automation techniques.
Companies can start small and do not need to seek perfection from the outset. Algomarketing’s data modeling roadmap provides a pragmatic approach to getting started, leveraging even the smallest amounts of data to build confidence and demonstrate impact.
This paper, the second in our ‘Future of B2B Marketing: Navigating the Algorithmic Landscape’ series, explores the opportunities available to every global enterprise business by looking at how best to approach data to realize the benefits of algorithmic marketing.
Practical advice includes:
- How competitive enterprises are recognizing the value of increased automation investment
- The AI technologies that can benefit marketers
- Realizing the value of your organizational data
- Data maturity issues impacting B2B enterprise marketing operations
- The Algomarketing data modeling roadmap
- Navigating data governance
- Corporate data and AI ethics committees
- The impact of corporate culture
Other articles in the series include:
- Demystifying algorithmic marketing for B2B enterprise: A guide to AI-powered growth
- Addressing the B2B enterprise marketing AI skills gap: building a sustainable operating model for the future
- Training the building blocks of optimal algorithmic marketing: tools, platforms and integrations for automated workflow optimization
- The AI maturity curve in B2B enterprise marketing: assessment, access and progression
- Ethical AI in marketing operations: mitigating tomorrow’s risks
- AI fears and hallucinations: maintaining human control through robust interfaces
- Democratizing AI: the two halves of algorithmic marketing and our solution
How competitive enterprises are recognizing the value of increased automation investment
With the current economic and business landscape characterized by uncertainty, it’s not surprising that many B2B enterprise marketing leaders are turning to automation techniques as a means to deliver efficient growth.
These tools and techniques not only enable growth but are realizing a future of work where humans can focus on strategy, creative ideation, and purpose because they are freed from the monotony of repetitive and time-consuming tasks.
From a technological perspective, MarTech solutions are already well-advanced, and widely available on the market at competitive prices. However, several constraints and concerns are holding large B2B enterprise marketing operations back.
Firstly, human skills capacity is struggling to keep up with the technology, with the battle to attract and retain digital talent the most competitive it has ever been. Meanwhile, concerns around trust in data, finding use cases, demonstrating value, and ethical considerations, are creating added hesitancy among senior leaders and key business decision-makers which is causing implementation delays.
The desire for clean and complete data is holding enterprises back unnecessarily. With the right expertise and tools, data can be trained and quality-built, starting with even the smallest incremental trials with a training data set.
Delivering AI-powered budget allocation for a tier one global tech brand reducing budget planning from months to days
By leveraging AI and machine learning, we built a recommendation engine to help decision makers at a global tech brand strategically plan marketing budgets to maximize ROI.
The model was trained with historical performance data across campaigns at scale, with business, macro, and micro-economic context. The tool was first tested as a pilot project in a specific region by A/B testing the AI-led versus the traditional budget allocation over a period of time. It was then scaled to other teams post-pilot.
Based on feedback, the model was fine-tuned to account for the organization's objectives and key results, targeting additional spend for specific regions to support their growth.
The tool enabled a more efficient budget planning process, reducing this part of the planning cycle from 3 months to a few days.
The impact of this was a 40% incremental uplift in marketing ROI versus the traditional method of budget allocation. Moreover, it enabled the team to uncover new opportunities and underserved audiences that drove higher returns.
Realizing the value of your organizational data
AI and advanced automation techniques have the power to transform marketing operations within large enterprises.
So what are the most common misconceptions about data?
The first centers around the idea that more data will provide a better understanding, or that quantity leads to better quality – improving accuracy through a broader view of the situation. The other big challenge is access. It’s not uncommon for data to have grown organically with a lack of clarity on where data is stored or who it is ‘owned’ by. It is often stored in silos and rarely available across the organization. These issues, along with a lack of in-house expertise to clean the available data, can lead to long-term IT data cleaning projects and reduce access to automation efficiencies.
Data maturity issues impacting B2B enterprise marketing operations
In addition to poor quality data concerns, enterprises are grappling with a range of data maturity challenges that can impact the effectiveness of their marketing activities. These issues vary across digital native and non-digital native brands.
Within non-digital native brands many challenges center around human capability and limited data literacy, often stemming from a resistance towards becoming a data-driven culture. This is only heightened by a lack of understanding regarding where data is stored, who owns it and how to access it.
Within digital native brands access to high-quality and bountiful datasets is less of a problem, but the value of these assets can be limited without sufficient use of advanced analytics. By not making use of key tools such as predictive modeling, machine learning algorithms, deep learning, and business process automation methods, organizations can be constrained in their ability to make data-driven decisions in real time. In turn leading to delayed access to deeper insights on market trends and customer preferences.
Likewise, insufficient data integration can prevent enterprises from seeing the bigger picture. For those with mounds of data at their disposal, it is important to become familiar with the process of combining data from different sources into a single, unified view.
The Algomarketing data modeling roadmap
The Algomarketing data modeling roadmap supports world-leading tech-driven enterprises in their ability to access and optimize algorithmic marketing techniques, regardless of data quality and maturity.
A key component of the roadmap is data annotation. A process concerning the labeling or tagging of data to provide context and structure and typically performed manually. It is crucial for supervised machine learning tasks, where models learn from labeled data with input examples and corresponding answers.
For instance, a B2B marketer could use data annotation to assign strategic importance labels (e.g. low, medium, high) to a subset of their accounts based on revenue potential, industry, engagement, and other factors. This would enable a model to be built to predict the strategic importance of new or unlabeled accounts, which would help the marketer prioritize high-value accounts, optimize resource allocation, and tailor marketing strategies, without the need to label data in the future.
Although not every dataset requires annotation or labeling, the practice is essential for building high-performing machine learning models.
Navigating data governance
No matter how an organization gathers, analyzes, and uses data, it will also be subject to various regulations depending on the jurisdictions they are operating in.
The two major markets with the most significant codes of conduct in relation to data are the European Union and the USA, the former launching its General Data Protection Regulation (GDPR) in the middle of 2018.
GDPR sets a high bar in privacy protection for individuals within EU member states, with laws applying to organizations that collect, store, or hold personal data belonging to data subjects. GDPR compliance requires adherence to a substantial set of rules that are based on seven key principles, including minimization in data collection, storage limitation, and accountability. Particularly sensitive data, including some health and financial information, requires extra protection.
The major difference between GDPR and US regulations is that the American data laws present a more fragmented picture. Different regulations cover different sectors and types of data, with specific regimes for financial institutions, healthcare organizations, and federal agencies. A handful of states have also issued their own sets of regulations, the most significant being the California Consumer Privacy Act (CCPA), which applies to consumers residing in the West Coast state.
Corporate data and AI ethics committees
Many organizations are now establishing a central data and AI ethics committee to help ensure it remains compliant within their jurisdictions. The remit of such committees is simple – to identify and help mitigate the ethical risks of AI products that are either developed in-house or purchased from third-party vendors. When AI and data solutions are proposed, it is the committee’s job to confirm whether they pose a serious ethical risk, recommend changes, or advise against adoption altogether.
Marketers must ensure they are fully prepared to present information regarding their clearly defined projects, data, data processing and access requirements, along with the anticipated business impact of how it will be applied to navigate these committees.
The impact of corporate culture
A data and AI ethics committee is indicative of an organizational culture that embraces data.
The role of an innovative, evolving culture in building trust in data and AI technologies cannot be understated. That culture must be bought into and embraced at every level, from the frontline of the marketing operations team, all the way up to the board and senior executives.
A data-driven culture is one that is based on a strong foundation of information and analytics. However, this does not mean that large quantities of data need to be collected before valuable insights can be derived or algorithmic benefits realised. In fact, cultures that are overly risk-averse in relation to data quality and quantity may miss opportunities to adopt transformative technologies and processes. Finding a balance is critical, and leaning too far towards caution can limit an enterprise’s competitiveness in the algorithmic revolution.
As with the issue of data quality and building trust in data, it is never too soon to start. The first steps can be small and experimental culminating in significant progress within your enterprise organization.
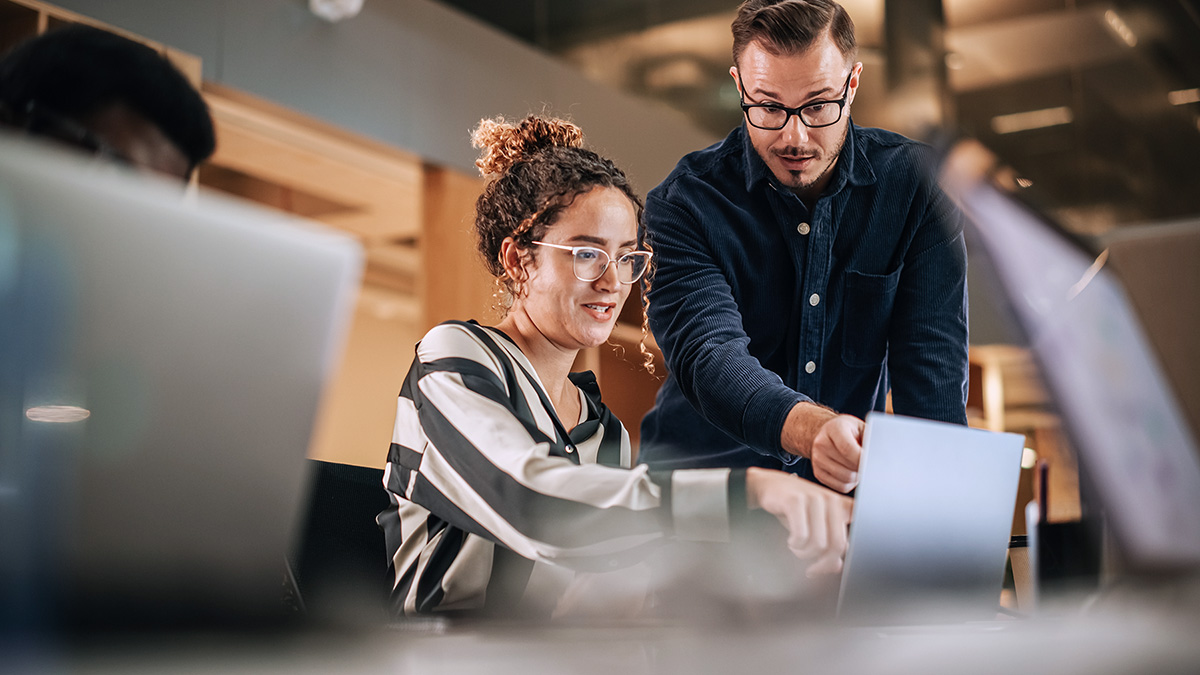
Conclusion
Concerns over data quality and privacy do not need to prevent organizations from investing in transformative solutions and techniques.
Algorithmic marketing is one domain where data maturity can be achieved over time, with companies able to start small and learn step-by-step, with guides such as Algomarketing’s data modeling blueprint providing a pragmatic approach to getting started.
Once the journey begins, there are maturity issues that will be encountered but providing enterprises and their marketing teams have the skills and expertise to manage them, these challenges are more than surmountable to accelerate growth through algorithmic marketing.
Algomarketing currently operates in 27 countries across six continents, using our unique methodology to unlock the power of algorithmic marketing through innovation, big data, and optimal tech stack performance for some of the world’s leading brands.
By harnessing the power of algorithms, we unlock incredible efficiencies, drive innovation, and scale operations like never before through AI, automation, and machine learning expertise. We empower marketing leaders to confidently make data-driven decisions, ultimately driving increased ROI.
Find out how we can help empower your organization today.
References:
- https://www.forbes.com/sites/forbesbusinesscouncil/2023/03/08/embracing-the-future-how-ai-is-revolutionizing-marketing-and-sales/?sh=676e3497bcc2
- https://www.mckinsey.com/featured-insights/artificial-intelligence/tackling-bias-in-artificial-intelligence-and-in-humans
- https://readwrite.com/is-your-data-good-enough-for-your-machine-learning-ai-plans/
- https://www2.deloitte.com/xe/en/insights/focus/cognitive-technologies/ai-and-machine-learning.html
- https://www.linkedin.com/pulse/tackling-marketing-automation-challenges-strategies-fearless-goetze/
- https://www.forbes.com/sites/cognitiveworld/2023/08/11/the-hidden-challenges-in-integrating-data-for-ai-systems/?sh=2488fcb459fa
- https://www.mckinsey.com/capabilities/growth-marketing-and-sales/our-insights/data-driven-marketing